Educators should approach early warning systems thoughtfully and with caution.
The field of predictive analytics — using data to predict future events — is growing in popularity well beyond education. Schools use early warning systems to anticipate which kids are likely to drop out of school. Market traders use data to forecast which stocks will increase in value, and police departments hire companies to determine where crime is likeliest to happen. Predictive analytics has even been showcased in “Moneyball,” a book and film celebrating data practices used by the Oakland Athletics baseball franchise to forecast which players would flourish, a strategy that helped the team beat the odds. Education is just one of manifold institutions using data to see the future.
There’s just one problem: As is so often the case with reform tools taken from industry and applied to schooling, there are differences between predictive analytics in education and other fields that are often ignored. Perhaps most important, teachers already know a great deal about their students — far more than an investor knows about a stock or a baseball scout about an up-and-coming pitcher. In fact, teachers are a veritable treasure trove of data on student behaviors, attitudes, and aspirations — information not typically included in a statistical model. Teachers also have far more power to shape what happens to students, an influence driven in part by their opinions of each kid. Using predictive analytics in education while ignoring these differences may lead to misidentifying students as at risk of dropping out and negatively influencing how teachers view those students.
Early warning systems in education
Broadly, early warning systems (EWS) involve two steps. First, educators use student data to predict which students are at risk of dropping out or experiencing some other outcome. Second, educators build systems of interventions and supports around the predictions to try to get the student back on track. Fundamentally, EWS are meant to thwart the very predictions they make. Currently, school districts and states employ such systems in an attempt to more accurately target scarce resources to students who need them most.
These predictions rely on using student data known to be precursors of dropping out. Much research documents which student data are the most accurate indicators of failing to complete high school. Unsurprisingly, students who eventually drop out often have low grade point averages, poor attendance records, and discipline issues. In some districts, these models are quite intricate. For example, certain school districts use statistical models to weight the different indicators according to how strongly they forecast dropping out. In others, the models are quite simple. For instance, educators might pick two or three indicators, use them to rank order students, then come up with an initial at-risk pool.
Of course, identifying this pool of students is only the beginning. What should schools do after the forecasts are made? For one, the prediction can help teachers generate conversations that improve the strategies used to support those students in the classroom (Allensworth, 2013; Davis, Herzog, & Legters, 2013). For another, predictions combined with student supports like targeted instruction and relevant professional development, including strategies for boosting student engagement, have been shown to improve the likelihood that identified students will graduate (Balfanz & Boccanfuso, 2007). Altogether, this research suggests that EWS are only as good as the conversations they generate and the actions to change students’ trajectories they ultimately inspire.
Despite this body of research, uncertainty remains about how, exactly, to use these systems to improve student outcomes. As a result, districts and states may develop EWS without first having a clear purpose beyond identifying students. My research and that of others shows how employing EWS without a concrete objective in mind can lead to wasted resources. Even worse, without a well-developed strategy for how to talk about students identified by EWS as at-risk of dropping out, school systems may inadvertently label students in a way that reinforces, rather than discourages, the forecasted outcomes. To avoid such pitfalls, research suggests educators should think carefully about the intended aims of EWS, as well as how (and if) information from those systems is shared with teachers, students, and other stakeholders.
Predictive analytics in education is unique
Many of the potential risks inherent in using EWS stem from ignoring or downplaying the facets of education that make it a different ballgame.
Early warning indicators are not early warning systems. For investors, predictive analytics begin and end with generating a prediction. Once a model has been developed for forecasting stock prices, the job of the system and its designers is largely done. By contrast, in education, predicting which students are at risk of dropping out is only the beginning. For this very reason, practitioners with experience developing EWS draw a distinction between early warning indicators (EWI) and systems (EWS): Indicators are the data points (like a student’s attendance patterns) that forecast dropping out; systems include the structures, supports, and practices designed to stop those forecasts from becoming a reality. Once the prediction is made, the job of educating begins.
Educators must define the theory of action in the beginning so it can inform the development of the early warning system.
While this point may seem obvious, there are subtler implications for how EWS — with an emphasis on “S” for system — are built. If the predictions can’t be decoupled from the actions they’re meant to generate, then educators must be very particular about which students they want to identify and why. For example, do teachers want to identify students who are most at risk for dropping out? Or are they interested in identifying kids who aren’t obviously off track but could be at risk of dropping out just the same? EWS could be designed quite differently depending on the answer. In short, educators can’t afford to let a statistical model determine how to proceed; rather, educators must define the theory of action in the beginning so it can inform the development of the EWS.
Objectives of EWS are often unclear. Despite the importance of establishing a plan of action, EWS could meet multiple goals, and these objectives can become muddled in practice. Among other purposes, one might envision an EWS being used to:
- Identify students who educators don’t know to be at risk;
- Confirm teacher beliefs about students they know to be off-track and help keep these kids on their radar;
- Generate predictions that are less biased toward certain student populations; or
- Monitor how many students predicted to drop outactually doso, either as a benchmark for success or accountability purposes.
Certainly, these objectives aren’t mutually exclusive, and there are other possibilities. However, the EWS developed and the costs associated with them might be very different depending on the intended use. For instance, an EWS would have to be much more sophisticated (using a statistical model, for example) if teachers want new information about students than if they simply want to generate predictions to organize teachers and encourage conversation around academic improvement.
Teachers are quite good at identifying off-track students. In stock trading, a broker knows something about companies and their stocks but is by no means an expert in the day-to-day operations of a business. By contrast, teachers observe students every day, and these interactions provide rich detail on student attitudes, behaviors, aspirations, and social proclivities. Moreover, such classroom-based “data” aren’t typically included in EWI. Though some school districts administer student surveys in an attempt to formalize these data, doing so is often costly, and measuring psychological aspects of schooling is difficult. As a result, most EWS rely solely on administrative data, including grades, attendance patterns, suspensions, and the like. Assuming that statistical models do better than simply asking teachers what they think will happen to a student may not be justified.
Research confirms as much. Studies show that teachers are quite good at predicting which students will drop out. In my work (Soland, 2013), 10th-grade teachers accurately predicted which students would drop out 89% of the time compared to 88% for EWI using statistical models. As one might suspect, teachers factored information on student attitudes and behavior into these predictions, which helped account for their accuracy. While more research needs to be done in this area, initial results show that EWI won’t always provide teachers with information they don’t already have.
Teachers have more power to influence predicted outcomes. Just because teacher predictions can be as accurate as model predictions doesn’t necessarily mean teacher judgments about students should be preferred. One reason to continue using EWS is that teachers have much more power to affect student attainment than a broker has to influence a stock or a talent scout the development of a baseball prospect. Bias in teacher predictions, which in turn can influence what happens to a student, may make EWS valuable, even if teachers accurately identify future dropouts, for a couple of reasons.
First, teachers may base their predictions on less than objective information. For example, I found that teachers were likelier to predict that Latino and African-American students would drop out (Soland, 2013). Even more troubling, these same teachers were likelier to mistakenly predict that minority students would fail to graduate. Whereas statistical models were also likelier to forecast that Latino and African-American students would drop out, there was a more even balance between wrongly predicting graduation and dropout for these groups. In sum, EWI and teachers were equally accurate, but model predictions were less biased toward negative outcomes for minorities.
Second, it is possible that EWI could change teacher opinions of students and not necessarily for the better. That is, EWI forecasts could become self-fulfilling prophecies: Labeling a student as likely to drop out influences teacher opinion, which in turn affects how the student actually performs. Though few studies investigate this possibility in the context of EWS, dozens of education studies find that self-fulfilling prophecies exist and that they have real if modest effects on student grades, attainment, and the like (Jussim, Eccles, & Madon, 1996). The most famous study is Pygmalion in the Classroom by Rosenthal and Jacobson (1968). In their study, the authors randomly assigned students to a group they designated as late bloomers. Without telling participants the labels were random, the authors shared them with the students’ teachers. Later, Rosenthal and Jacobson revealed that students labeled as late bloomers had worse outcomes, suggesting teacher reactions to these labels drove the results. Surprisingly, no research considers the possibility that EWS and the ways in which they label students may be producing a Pygmalion effect.
No work has been done to combine human and statistical forecasts. In other professions, much work has been done to understand not only when to use data-based versus human predictions, but also when to combine them. Such combinations can allow users to capitalize on professional judgment while safeguarding against bias and incorporating the seemingly limitless information made possible by computers. For example, in medicine, research shows that the most accurate diagnoses of health conditions are often made when computers use symptoms to sift through thousands of known ailments, then doctors use those matches to inform their professional judgment. (McClish & Powell, 1989). Beyond medicine, these human-data hybrids appear most justified when people have information not easily included in datasets. In short, education seems a perfect fit for combining human and EWS forecasts, but no research suggests how to do it.
Being strategic about EWS
School systems don’t have the time or money to invest in innovations that fail to provide actionable information or improve practice. Educators tasked with improving outcomes for underserved communities especially can’t afford to implement a reform that doesn’t improve achievement or is a detriment to it. Though research shows EWS can help organize teachers around low-performing students in a way that reduces dropouts, these systems could just as easily waste resources or bias teacher opinions of students. The difference between these two possible realities boils down to how thoughtful districts and states are about these EWS, especially matching the system to what they hope to get out of it.
Though more research is needed on EWS and their uses, a few lessons present themselves on how to be strategic about designing them. First and foremost, Early Warning Indicators only become Early Warning Systems when educators have built supports and interventions for teachers and students around the predictions in a meaningful way. Second, such systems are most effective when districts and states start by knowing which students they want to identify, what information they hope to glean about those students, and how they will match those kids to meaningful academic supports. Finally, the policy makers in charge of adopting and implementing EWS should think of teachers not only as interpreters of data but also as valuable sources of it. Sometimes, data can be used to reinforce teacher professional judgment. In other cases, it can serve as a check to ensure that decisions about students aren’t being made too subjectively. Either way, the intended use drives how the EWS should be built — a lesson that’s all too easy to forget.
References
Allensworth, E. (2013). The use of 9th-grade early warning indicators to improve Chicago schools. Journal of Education for Students Placed at Risk (JESPAR), 18 (1), 68-83. doi:10.1080/10824669.2013.745181
Balfanz, R. & Boccanfuso, C. (2007). Falling off the path to graduation: Middle grade indicators in [an unidentified northeastern city]. Baltimore, MD: Center for Social Organization of Schools.
Davis, M., Herzog, L., & Legters, N. (2013). Organizing schools to address early warning indicators (EWIs): Common practices and challenges. Journal of Education for Students Placed at Risk (JESPAR), 18 (1), 84-100. doi:10.1080/10824669.2013.745210
Jussim, L., Eccles, J., & Madon, S. (1996). Social perception, social stereotypes, and teacher expectations: Accuracy and the quest for the powerful self-fulfilling prophecy. Advances in Experimental Social Psychology, 28, 281-388.
McClish, D.K. & Powell, S.H. (1989). How well can physicians estimate mortality in a medical intensive care unit? Medical Decision Making, 9, 125-132.
Rosenthal, R. & Jacobson, L. (1968). Pygmalion in the classroom: Teacher expectation and pupils’ intellectual development. Norwalk, CT: Crown House Publishing.
Soland, J. (2013). Predicting high school graduation and college enrollment: Comparing early warning indicator data and teacher intuition. Journal of Education for Students Placed at Risk (JESPAR), 18 (3-4), 233-262.
R&D appears in each issue of Kappan with the assistance of the Deans Alliance, which is composed of the deans of the education schools/colleges at the following universities: George Washington University, Harvard University, Michigan State University, Northwestern University, Stanford University, Teachers College Columbia University, University of California, Berkeley, University of California, Los Angeles, University of Colorado, University of Michigan, University of Pennsylvania, and University of Wisconsin.
Citation: Soland, J. (2014). Is “Moneyball” the next big thing in education? Phi Delta Kappan, 96 (4), 64-67.
ABOUT THE AUTHOR
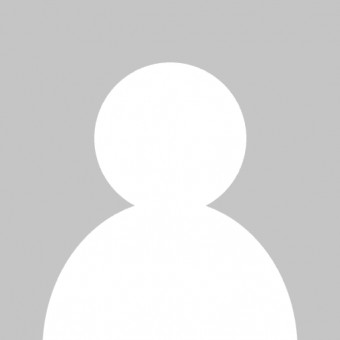
Jim Soland
JIM SOLAND is a doctoral student in developmental and psychological sciences at Stanford University, Stanford, Calif.